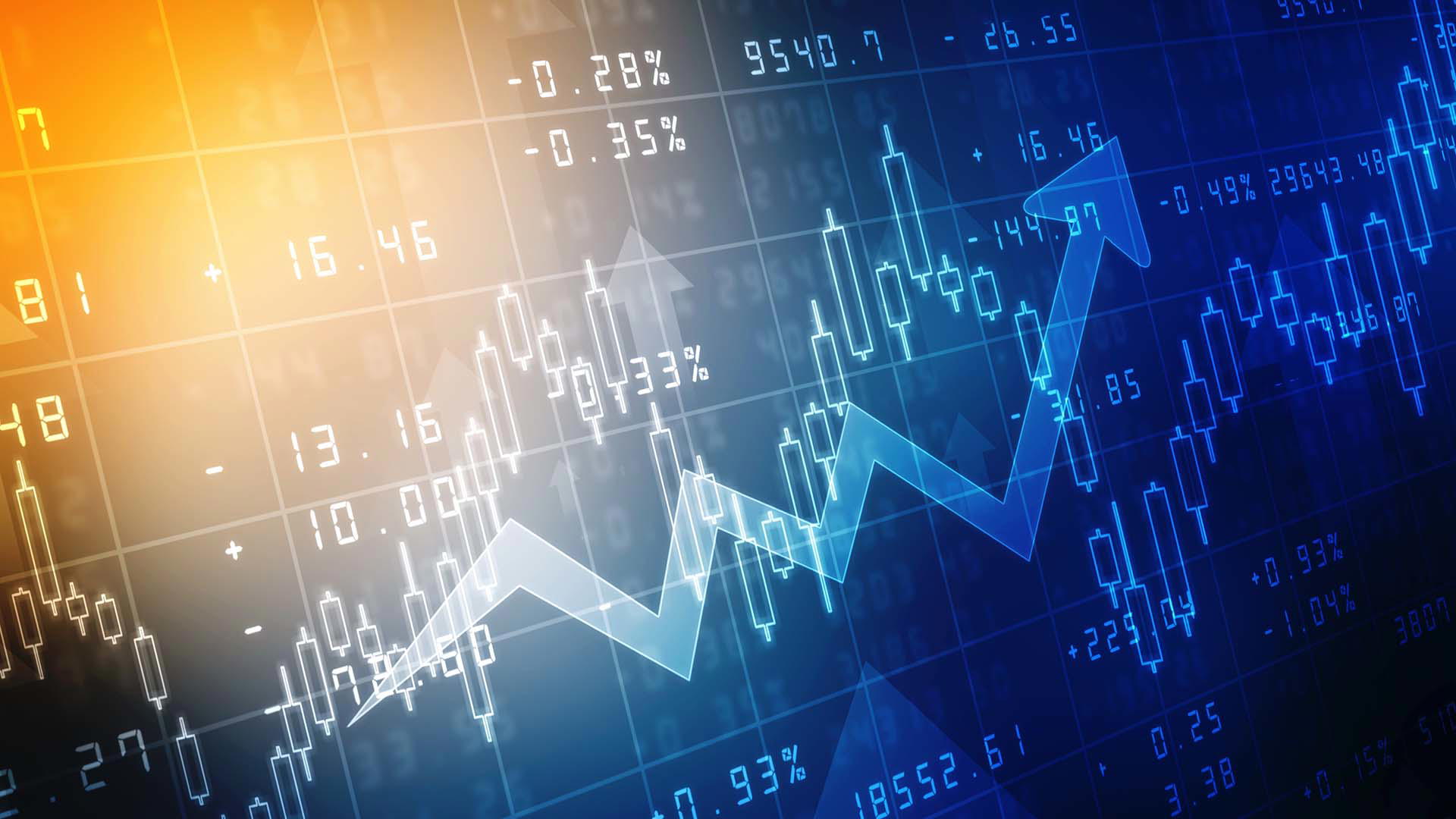
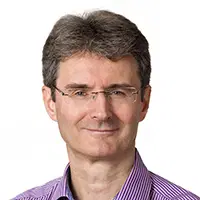
- Tom Alford
- Deputy Editor, Treasury Management International
Cash forecasting accuracy is central to the corporate treasurer’s capacity to optimise liquidity in a highly volatile macroeconomic environment. TMI calls on a brace of expert witnesses – Ed Barrie, former treasurer and now Co-Founder and Chief Product Officer, Treasury4, and Dr Hui Gong, Lecturer in Fintech, Westminster Business School – to examine the most effective approaches to forecasting.
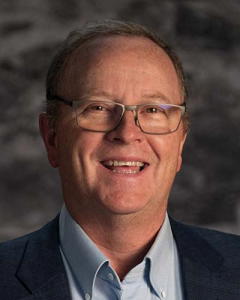
Ed Barrie
Former treasurer and now Co-Founder and Chief Product Officer, Treasury4
Cash flow forecasting accuracy is a treasury staple, generally sitting within the top three of the hierarchy of treasury needs. But it will quickly become the number one priority during times of economic uncertainty, when increases in interest rates, periods of business stress or M&A activity are heightened.
This is outcome shown quite clearly in results from the EACT Survey 2022 (see chart 1) which notes “cash flow forecasting and treasury digitisation are likely to remain [treasury] top two priorities over the coming 12-24 months”.

As a dynamic point of interest, Barrie notes cash flow forecasting tends to go through periods of intense focus inside of the organisation, especially if executive compensation is tied to measures such growth of free cash flow. “No CFO or treasurer is ever fully satisfied with their cash flow forecasting process. There is always room for improvement, especially when tapping into underlying source data from ERPs and line of business systems.”
For those who feel they are not getting the right results, the prospect of new technology is compelling. But cash flow forecasting is more than a technology issue, cautions Barrie, adding that “many organisations have not defined exactly what they want to measure in terms of a forecast”.
If this is the reality, then the first step is defining what the organisation wants to measure. To do this, the following questions must be addressed:
- Is your organisation looking to forecast operating, financing and investing cash flows along with key measures such as operating cash flow and free cash flow? Or is the organisation trying to obtain a more granular forecast of AR/AP, payroll and taxes?
- Is this to be viewed by subsidiary level or even at the individual bank account level?
- Is forecasting at the organisation reporting currency level or at the transaction currency level?
- If forecasting is at a granular level, can it be rolled up to the operating, financing and investing level?
- What is the time horizon you want to forecast?
Traditionally treasury has focused on 13-week rolling forecasts while financial planning and analysis (FP&A) owns the long-term strategic cash forecast. Barrie encourages both teams to be actively collaborating to ensure that both forecasts support each other. “The starting point is to define exactly what your organisation wants to forecast and the time horizon, along with the key stakeholders and the team members responsible for managing the forecasting process.”
One of the keys to successfully collaborating on cash forecasting is the deployment of relevant technology. However, it must be accepted that technology is only as good as the inputs, including data that are fed into the solutions. “Many organisations have not created an inventory of underlying source systems that contain data – such as ERPs, purchasing and procurement, corporate card, merchant services, payroll, investment management, debt management and sales management,” comments Barrie. it’s important that organisations attend to this oversight..
Once the systems and data inventory (including any relevant manually sourced data) are available, then the organisation can start building the interfaces to source the required data. This can be fed into the forecasting solution to produce the forecast, and adequately measure the forecast versus actuals, using bank statement reporting to identify accuracy and sources of improvement.
“Forecasting should not be treated as a set it and forget it process,” Barrie warns. “Rather, it’s a continuous undertaking that needs to be adjusted and fine-tuned as the underlying business grows and changes, economic conditions change, and systems and people change inside the organisation.”
Indeed, he feels that the best treasury teams have forecasting embedded as part of their DNA and daily operations playbook, including “actively evangelising the benefits to stakeholders and team members throughout the organisation”.
The cutting-edge
Where new forecasting technology is appropriately deployed, the notion of what is advanced tends today to leverage ML, AI, data management and data visualisation tools. These will be deployed in a scalable and flexible way to present users with information.
“In context, ML technologies can be employed against historical bank statement transaction data to provide a projected distribution range for forecasted cash flows. These will likely be more effective than manual forecasting in spreadsheets,” explains Barrie.
This approach can be enhanced by introducing source data for all known contracted future cash flow related data. This list may include open AP and AR invoice level data, payroll, tax, debt and investments. “But use of ML is only as good as the data inputs, including model assumptions and the ability for the user to understand the measurements,” notes Barrie. “Users responsible for owning the forecast still need to validate the outputs for reasonableness, and be able to explain the forecast to stakeholders.”
Good forecasting tools should enable organisations to ingest large amounts of source data, preferably at the transaction level. They should then be able to summarise that data into the cash flow categories over the applicable time period, with the ability for users to drill down into the source data for deeper insights. In short, data should be presented visually to users and should surface cash flow trends and material cash flow transactions into which users may want to delve deeper.
The sheer volume of data that could be used may raise the threat of ‘analysis paralysis’. However, notes Barrie, this can be avoided by presenting users with distilled information, and not overwhelming them with data, while supporting flexibility for ‘what-if’ scenarios, seasonality, growth assumptions, and leveraging ML that factors in historical actuals from bank statement reporting to provide a distribution of forecasted results.
Solutions for all
In many cases, the benefits of cutting-edge technology may be purely academic because budgetary constraints may not permit the discussion to go any further. But Barrie argues that treasury departments can make lower-cost technology improvements by looking at cloud-hosted solutions versus on-premise solutions, and subscription-based services versus multi-year contracts. “Banks are providing in-house-built forecasting solutions as well as partnering with third-party fintech solutions, all of which open opportunities for treasury,” he adds.
There is one technology improvement that Barrie believes all treasurers should consider making, and that is developing team skills around data visualisation and data analytics, using industry solutions such as Tableau, Power BI or Google Analytics.
“These tools have very powerful and business-user friendly capabilities, and they are continually evolving with new features and functionality,” he explains. “Best-in-class treasury departments are also centres of excellence for data analytics for their organisations, and cash flow forecasting is the intersection of data and analytics.”
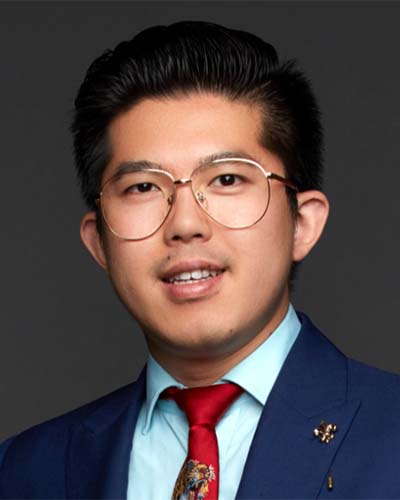
Dr Hui Gong
Lecturer in Fintech, Westminster Business School
Dr Hui Gong, Lecturer in Fintech, Westminster Business School explains how data techniques and technology combine to improve accuracy
Currently, the more effective method used to arrive at accurate cash forecasts is through historical data analysis. From a probabilistic point of view, cash flow is unpredictable because economic conditions change rapidly. For example, last year, you could not imagine the impact of Russia’s war on Ukraine on the level of prices, especially the impact on raw materials and supply chains. But historical data can at least tell us what we need to do when a certain situation occurs to achieve a relatively large win rate.
Due to the surge in the amount of data, this kind of analysis has begun to transform from ordinary statistical methods, such as linear regression, which finds a straight line that minimises the discrepancies between predicted and actual output values, to ML technologies such as supervised learning, which use algorithms to update iterative parameters as the amount of data increases, thereby improving the accuracy of predictions.
At present, most companies are in a very early stage of using these techniques and tools in their cash forecasting. The exception is for some large-scale financial companies, which have the technical strength and aggressiveness – and budget – to do this. It will be difficult for most SMEs to invest heavily in cash forecasting at this level. As a result, the methods they use are still relatively basic.
Data first
For those who know they need to improve their cash forecasting, their first step to designing a new approach to gathering, analysing, and presenting forecast data should be to build their own database to collect relevant data. The source can be the company’s own data, but they will also need to bring in economic data, especially macroeconomic data, to incorporate the unpredictability of current and ongoing conditions.
It is essential that sufficient data is used, otherwise the analysis will be biased. At the same time, I suggest trying several methods of analysis, especially the methods used in supervised learning that enable regression algorithms to map a relationship between input variables and output variables.
There are many methods that can be tested to reduce prediction error, such as the stress test – which is a simulation technique to evaluate how forecast results might react in various situations, and the robust test – which can test if forecast assumptions are true and can give valid results across a variety of conditions. Each type of supervised learning usually has its own corresponding testing methodology, but the purpose is always to be able to use the data to answer questions, and maybe uncover hidden truths about that data set.
In terms of what treasurers should realistically expect from using the latest approaches to forecasting, I’d say that most treasurers are likely to be more concerned with trends and coping strategies after extreme events. As for predictions, they may not care about accuracy so much, but they do still need to know if a trend is positive or negative, and whether or not the prediction method being used remains effective after a major event occurs.
The way treasurers can be sure they are taking the right action is simply to make adequate preparations and strategies. So even if they achieve a more positive forecast, they need to prepare Plan B to deal with financial constraints as these may arise at any time.