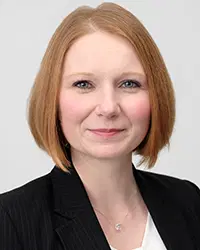
- Eleanor Hill
- Editorial Consultant, Treasury Management International (TMI)

- Gautier Mouzelard
- Head of Transformation Programs, BNP Paribas Cash Management
What does the rise in popularity of AI mean for corporate treasurers? Eleanor Hill, Editorial Consultant, TMI, recently spoke to Gautier Mouzelard, Global Head of Industrialisation for Trade Finance, Acquiring and KYC, BNP Paribas, to assess the potential implications while outlining how treasurers could approach using AI in their day-to-day processes.
Eleanor Hill, TMI (EH): AI is not a new technology per se, but people are suddenly talking about it as if it’s a groundbreaking development. What’s driving the current momentum, and do you think AI is overhyped?
Gautier Mouzelard, BNP Paribas (GM): We already use AI in our day-to-day life in different ways, but I’d describe this usage as being in ‘stealth’ mode. We use AI to unlock our mobile phones through biometrics, for example. Or when we see product recommendations on online marketplaces, those are driven by AI based on our previous buying behaviours and browsing habits.
There are a couple of drivers for AI’s current prominence, the first of which is the recent release of ChatGPT, a type of generative AI. This tool has gained significant notoriety and has quickly demonstrated the potential of AI to the general public – in a very tangible and accessible way. It shows the opportunities the technology holds and links to the second driver, the evolution of AI technologies. Together, these two strands highlight what’s possible with AI for consumers, but also for corporates and banks.
EH: Do you think that generative AI is the most exciting type of AI, or are there other, more innovative developments treasurers should be investigating?
GM: Generative AI is definitely the most promising type of AI that will have substantial benefits and could lead to deep changes for banks and corporates. However, generative AI is not yet widely used in our industry – and that’s not only down to the technology. There are challenges to address regarding reliability of data and approaches to data sharing, which naturally carries the need for great rigour and scrutiny.
Machine learning [ML] and natural language processing are other aspects of AI that have already proven their worth and will continue to bring considerable value in multiple use cases. These are extremely exciting and powerful in the context of AI and many tools we use on a daily basis already leverage these capabilities.
EH: We know there are many potential benefits of AI, but what are the primary downside considerations – such as ethical concerns or potential job losses?
GM: AI is an evolving technology, and all technologies face these kinds of questions in the development phase. When it comes to the best and worst aspects of any technology, it depends on the regulation and also the organisation’s appetite in terms of where its boundaries are. If you take the example of the internet, it’s the same story – making it work for the company depends on internal controls and boundaries, as well as defining specific use cases and user rights or privileges.
Looking from a banking perspective, I would say there are three main considerations around AI. The first is data dependency – we need a vast quantity of data for the optimal use of AI. That’s not always easy to source or manage. Actionable AI outputs also depend on the quality of the data that is inputted. If you do not have reliable data or qualitative data, the AI won’t add value or, even worse, will impact process quality. So, it’s critical to have a robust approach to data in place in order to derive the desired benefits.
The second challenge is the cost of AI. Of course, there is a direct cost, but there are also underlying overheads to be aware of – and this can be hard to quantify. For example, the right technology and data architecture needs to be set up, and then constantly maintained and upgraded, in order to enable the AI to function fully. There are also human resource outlays to consider since data scientists and experts will be required to design and create the AI ecosystem. Then there is the cost of people to control the AI once it is up and running. Experts in this field are at a premium, so it’s not a cost to take lightly.
Finally, the third challenge is the change management aspect, especially given that the use cases for AI will grow and change. And as banks progressively move from leveraging AI for basic operational tasks to more advanced analytics tasks, change management will be vital. In large organisations like banks, people will have to be specifically trained for this shift.
EH: Given the challenges, why is it a priority for BNP Paribas to embrace AI? Where do you see it bringing value?
GM: As a financial institution operating on a global scale, AI holds the potential to complement the existing services we offer to clients worldwide via enhanced data analytics. What’s more, we are very well positioned to get the most out of AI since we hold significant amounts of processes, client interactions and accurate data from transactions that we process on behalf of our clients. For BNP Paribas, a bank that prides itself on innovation, any technology that can process this large amount of data and derive deeper insights must be leveraged.
AI also brings the potential for significant efficiencies, meaning that the bank can leverage it to deliver an even better customer experience and an improved employee journey, especially around informed decision-making. With its ability to spot outliers, AI can also help to protect the bank and our clients when it comes to fraud mitigation and AML.
EH: Is there anything that treasurers could be doing now to take advantage of AI rather than simply leveraging what the banks and TMS vendors are working on?
GM: For treasurers, in the short term it’s all about simple process improvements and eliminating repetitive manual processes, freeing the team up to focus on more strategic tasks. Then, in my view, one of the most fascinating use cases is for improving cash flow forecasting processes and accuracy. AI can bring considerable value to treasury decision-making here through instant scenario planning and analytics, for example. Outside of forecasting, there are clear use cases for processes such as automated bank reconciliation and the prevention of fraudulent or duplicate payments. But speaking with banks and tech vendors can certainly be helpful here, as often they already have these kinds of AI-driven solutions available.
EH: For those treasurers who haven’t thought about AI or don’t know where to start, how should they approach embarking on an AI project?
GM: The critical first step is to determine a use case with a clear need. Identify where AI could help, don’t look at AI first and your processes second. Define the main processes in the value chain and exactly where the data gaps or inefficiencies sit. These are typically the areas where treasury could add analytics or automation, for example. Also examine where treasury may want to reinforce controls or reduce simple, repetitive tasks. In short, the starting point is to step back, critically re-assess your current workflows, pinpoint what isn’t working as well as it could, and then look at the market to see the solutions associated with that need. Also be aware that AI might not always be the right answer! There are other tech solutions out there which could be a better fit. So, it’s important to keep an open mind.