Since ChatGPT was launched by OpenAI in November 2022, it has been touted as a potential solution to many business challenges. So, could advanced digital technology such as generative pretrained AI help to enhance the capabilities of corporate treasury functions?
First, let’s look at the fundamentals. ChatGPT (where GPT stands for Generative Pre-trained Transformer) is a generative pretrained AI-based chatbot which has whipped the corporate world into a frenzy over the past few months.
As the name suggests, GPT technology can generate entirely new content – from natural language to code, and text to voice. This could be used for everything from creating art to writing marketing materials. ChatGPT is also helping students answer university-level questions, write programming codes and writing essays which – to all intents and purposes – appear to be drafted by human being. What’s more, all of this can be done in seconds.
But could GPT AI technology be successfully applied to benefit treasury functions? We think ‘yes’, this could increasingly be the case ¬– but only in certain and areas, and caution is definitely required. And perhaps it is the offerings from financial institutions to treasury functions which will be more directly impacted, certainly in these early days.
Re-framing treasury interactions
To better understand the potential of ChatGPT, let’s reimagine various aspects of treasury functions through the lens of generative intelligence. It is common knowledge that treasury management has always been a key finance function that ensures optimal utilisation of resources/capital combined with efficient risk management within the regulatory frameworks. Treasury also works on maximising the return on investments, while minimising costs where possible (since many treasuries are no longer cost centres, and instead are value creators).
The rapid digital transformation in banking and the financial services sector over the past decade has further increased the responsibilities of this core function and the pandemic has propelled treasury responsibilities to new heights. Treasurers are now involved in everything from digital technologies and new distribution networks to digital assets, CBDCs, and NFTs – all while fighting cybercrime and fraud, embracing instant payment and collections, and keeping up with regulation across the globe.
In addition, the current higher interest rate regime fuelled by inflationary trends further increases the need for corporate treasury to develop capabilities for efficient capital deployment and risk management – especially as M&A ramps up. This creates a renewed need for digital technology intervention to support decision-making, human machine interaction, automated risk monitoring, and alerts to name some.
At this level of digital transformation, AI is one of the foremost technologies able to offer significant wins. And in recent years AI has come a long way from chatbots to fraud monitoring and product customisation. Generative AI is the latest trend and could potentially be used in the following applications impacting treasury:
Improved virtual banking experience
Managing bank relationships and quickly extracting data from banking partners is an ongoing challenge for corporate treasurers. As bank branches have closed, due to the rise of online banking and the pandemic, digital assistants have started to fill the gap. But as many will attest, in the early days these chatbots were constrained by limited content – and certainly not helpful for corporates with complex needs.
Now comes ChatGPT, providing human-like conversation, which has the capabilities to provide the missing element to corporate-bank conversations. In time, we envisage that this could be a game-changer in customer service management within corporate banking, especially where large volumes of focused queries are handled. This could provide an add-on to self-service tools for payment tracking, for example.
Hyper-personalisation in investments and derivatives
Treasury departments often deal with the full spectrum of investment solutions from deposits to MMFs, while managing interest rate-, FX- and commodities risks via derivatives. Banks and asset managers already deploy AI and ML to customise investment solutions, especially in the retail space, and there is no reason why this could not trickle through to treasury investments, and FX hedging, for example.
Today, ChatGPT is opening up the possibility of creating hyper-personalised products using generative AI technology in seconds, while in conversation with customer. With its power to interpret and summarise customer requirements, combined with additional profile intelligence and selective human touch, this could usher in an era of more targeted investment solutions and derivative products for corporates – especially as other trends such as tokenisation take off.
Improved risk assessment capabilities and deployment of capital
Technologies such as DLT have led to the evolution of digital assets, while fast-tracking the movement of money across the globe at an astonishing speed. For some, especially retail investors, digital assets created huge opportunities. But for others, the associated risks have proved too significant to ignore.
The good news is that ML-powered generative intelligence is expected to provide superior capabilities to assess risks in areas such as digital assets, and monitor fraud threats continuously, using higher computing capabilities.
With these transformative abilities, we expect to see technology create on-demand scenarios that can enable treasury functions and financial decision-makers to make more informed choices in real-time around the deployment of capital – outlining both potential upsides and downsides. Interestingly, as well as financial capital decisions, AI could also be used to determine how to use employee-, intellectual-, and social capital more efficiently.
When it comes to capital market decisions specifically, AI could also help to increase certainty. Currently, most of the methods used in analysing capital market phenomena are based on descriptive statistics, with a foundation in classical methodologies. Researchers are now focusing more on identifying the fine line between knowledge and certainty.
Knowledge is merely a repository of different scenarios and its outcomes. So, the question is whether this collection of scenarios is all-inclusive? If the answer is no, then there is gap in understanding different dimensions comprehensively, and a lack of certainty. This is where data can aid in accessing insights by extrapolating the observed values – and generative AI powered with ML models and algorithms can create scenarios, simultaneously challenging and improving existing frameworks.
Possibilities of GPT in treasury
In order to leverage a ChatGPT-like model in treasury functions, in addition to large language models, it is necessary to define a domain-specific foundational model that is trained on financial principles, concepts and various asset classes. The model should be trained on fundamental and technical evaluation techniques, across a wide range of companies and industries, as well as nuances of complex financial instruments, associated risks, regulatory requirements, market behaviour, and so on, to develop capabilities that create products and portfolios suited to the corporate’s investment profile and policy.
In addition, the model should be capable of decoding changing regulations, assessing the impact of these shifts and accordingly re-evaluating the portfolios and processes. All of these are necessary inputs when creating a foundational model for treasury operations. The combined language plus financial domain model could then provide insights on a market or instrument and aid complex investment and business decisions.
Enormous investment
However, creating such a model is a colossal and complex task. In addition to the know-how and resources to develop it, the model would also require large amounts of high-quality clean data and a domain-specific taxonomy. Multiple iterations of fine-tuning would also be required to learn the specific vocabulary and structure, and to be able to effectively predict and perform tasks specific to the domain.
In the event that such a financial foundational model is built to assist a sophisticated treasury function, there should still be a mechanism to include a human in the loop, since every model has its own biases, and can lead to potentially disastrous outcomes, if it draws on misinformation. The human in the loop will not only aid the process execution, but actions and decisions taken by the human will also feed into the reinforcement learning mechanism of the model.
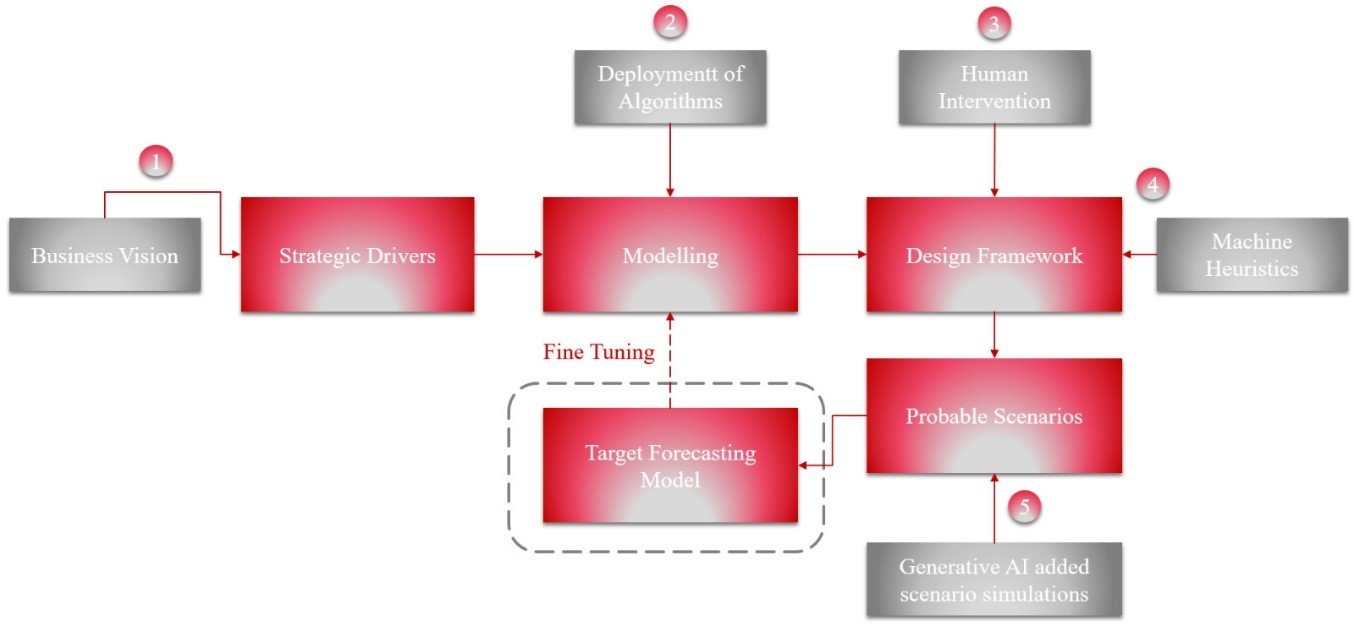
Please click on the image to view a larger version
In strict terms of STP, the human in the loop could be considered as a probable frailty of an exhaustive cognitive approach. However, by embracing a mid-way, human-centric cognitive approach that is precisely based on an interactive model, value can be derived by augmenting the interactions between human and machine, with the human brain acting as another computational intersection.
For example, the machine may add value using unrivalled memory power and impeccable computational capabilities that is required to determine pricing of securities accurately, however the human brain can accentuate the interactions using heuristics – like model selection based on beta regression for ML systems, which needs human supervision. That’s how the efficiency of future markets may be enhanced through a hybrid approach. Some researchers have already demonstrated that if we try to explore the best price for securities in the capital market, combined human brain and machine interactions can reduce the convergence time compared with the classical cognitive approach.
Staying grounded
Like any nascent technology, generative intelligence is still developing – and therefore has risks attached. And as with any other AI model, it is based on the underlying algorithm and the limits of the data that the model is trained on. In addition, it has its inherent biases. It is therefore advised to exercise caution around GPT, to understand its capabilities and limitations. While offering many data elements to explore, experiment, and harness the transformative power of this new technology, treasury functions are sensitive and conservative by nature – not the natural home for cutting-edge experimentation.
As Sam Altman, Co-Founder, and CEO of OpenAI warned during a venture-capital-focused event in San Francisco in January: “I think the good case [for AI] is just so unbelievably good that you sound like a crazy person talking about it. I think the worst case is lights-out for all of us.”
About the authors
Debanjana Dasgupta is a Senior Architect in a leading technology MNC. In her latest role she advises clients on their digital transformation journey with a sharp focus on process excellence with intelligent automation. A passionate writer, she is a published author of the book Intelligent Automation Simplified from BPB publications. She is based in Delhi, India and can be reached at [email protected]
Manas Panda Ph.D is a partner in a leading technology MNC advising banks and financial institutions on implementing their digital transformation strategies with focus on customer experience and operational efficiency. A Stanford LEAD alumnus, he talks about technology innovations in financial services. He is based in Toronto, Canada and can be reached at [email protected]
Raja Basu is a Senior Consulting professional in a leading technology MNC. In his current role he works as a business architect and helps clients to enable their digital transformation journey. He has special interest in responsible use of AI. He is also pursuing his doctoral studies (Ph.D) from XLRI Jamshedpur. He is based in Kolkata, India and can be reached at [email protected]