AI is now part of everyday life. As a result, we have a technology – and one that is being continually improved – that helps us to perform tasks more efficiently. But we also want from AI is a system that will not interfere with or undermine human capabilities.
One of the biggest challenges around the development of AI has been to set appropriate boundaries because an AI system can potentially lead to catastrophe unless it is controlled within limits. Despite all the benefits of AI, there are concerns specific to the ethical aspects of AI systems.
Of all the considerations, climate change is undoubtedly the most important, at least in my opinion. The impact the climate has on every aspect of modern life (and vice versa) now has the full attention of governments and corporates. At a holistic level, we can see that the weather phenomena the world is experiencing, from floods and hurricanes to droughts, are proof that these are the results of interconnected intervention, which we can safely refer to as human-induced climate change.
Invariably these phenomena are triggered by the use of technologies (and machines) that generate excessive greenhouse gas (GHG) emissions. Our zest for building AI systems and championing cognitive modelling is slowly but steadily propelling us towards to a ‘new world’ – where we are compelled to become more conscious about ESG-related issues in a bid to reduce the overall health of the global ecosystem. Although many are saying this wake-up call is already too late.
What’s more, all of the ESG efforts being made today may be just a drop in the ocean – and this is where the ethical aspect of AI comes under the spotlight. We now must focus more on striking the balance between the ‘need’ and the quest to satisfy it. In addition, we must be aware of the outcomes of AI-enabled decision support systems, and whether they are equitable.
The challenges of AI ethics
In other words, it is essential that we design, build, deploy, and make use of AI in an ethical manner. Indeed, according to the IBM Global AI Adoption Index 2021, 91% of businesses currently using AI think that being trustworthy and explainable is critical for driving the business seamlessly. But building ethical AI is not easy. It requires a multidisciplinary approach in that it tries to identify the process of optimising AI’s beneficial aspects while eliminating risks and adverse outcomes. The diagram (fig. 1) below sets out the key elements of AI ethics.
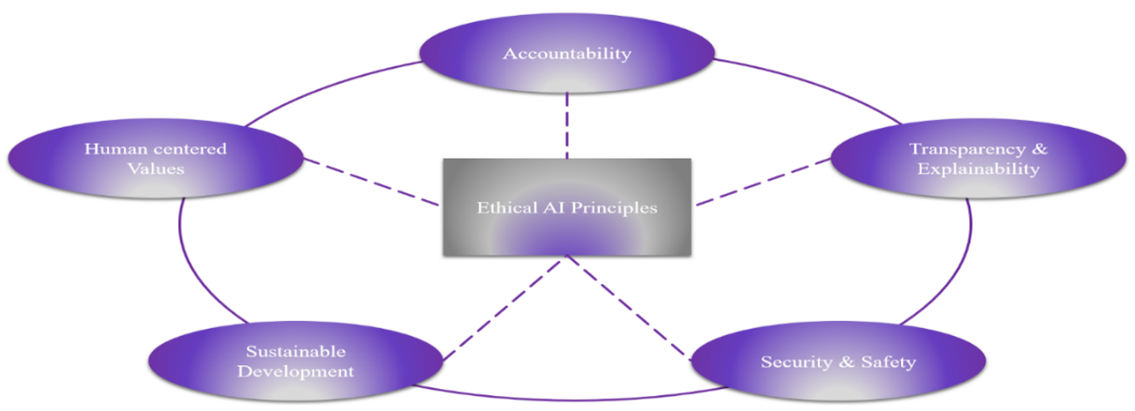
Figure 1: Different dimensions of ethical AI
Security and safety: AI systems should be technically sound and robust in order to withstand malfunctioning and external hacking. As we know, autonomous systems have become more common in society today. Therefore, it is critical that the systems are robust and operate as intended. In the same way, the development of autonomous trading systems needs to be able to offer assurance that strong robustness guarantees can be made (Weld and Etzioni 1994).
Transparency and explainability: This feature is vital towards developing trust surrounding AI applications. This has been predominantly highlighted by researchers that several factors are critical that help to understand the attributes of better comprehensibility of an AI system, including transparency, traceability, and interpretability (Grzymek & Puntschuh, 2019; Koene et al., 2019; Rohde, 2018). Those building AI systems must try to design those to be as transparent as possible, these are often referred to as explainable AI. And plenty of literature is available that focused on examining new methods of interpretability (Guidotti et al., 2018; Samek, 2019).
Accountability: Businesses should be accountable for the data, as the rationale suggests that the data-specific insights belong to the owner of the data. Hence, whatever decision emerges, the onus should lie with the data owners. Systems should also have explainability features. Plenty of biases occur due to human interventions and absence of proper accountability. Psychological studies suggest that humans have a tendency to make decisions subconsciously and then to further consciously rationalise the same later (Soon, Brass, Heinze, & Haynes, 2008).
Sustainable development: There must be a sense of wellbeing for the end users, and that can come only from trust and fairness. This has a profound impact on associated ESG ecosystems. It has been mentioned that end users’ demand is continual, and that also adversely impacts ESG wellbeing (Csikszentmihalyi 2000). A sense of security and trust can help when constructing a pragmatic sense of awareness. The aim is to have a holistic impact assessment, carried out by the centre of excellence (CoE) team within the host organisation so that the use of exponential technologies will not impact society and our larger social ecosystem. CoE will also evaluate the need of adoption and scale related issues. These should be done as a regular interval may be half yearly depending upon the size of the organisation and the complexities of the applications, all these must be incorporated as part of governance.
Human-centred values: AI systems should be developed and deployed in such a way that they will respect human autonomy and prevent any intentional harm; it should also display fairness and explainability. In case any conflict arises, it should be addressed with immediate effect. Special care should be taken to address vulnerable groups where asymmetric distribution is evident so that no preferential treatment is meted out to any specific group. This exercise will ensure homogeneity and uniformity are maintained. Human-centred design build on the principle of design thinking will enable to address this challenge to a large extent.
It is evident that the use of AI systems should be executed with great attention and a proper governance framework should be in place to take care of debiasing technique. AI governance can be construed as the process of defining policies and institutionalising accountability to guide the conception and deployment of AI systems in any organisation.
AI in treasury
Even today, AI is inextricably connected with the operational aspects of TMSs. Given the challenges and concerns outlined above, it is imperative to understand the paradigms where ethical vulnerability exists. Asset allocation and distribution is key to efficient treasury management, for example, and that also includes diversification and rebalancing – and these processes may well leverage AI now and in the future.
Here are some areas where AI can be used responsibly to achieve optimum output in the treasury function, and associated departments
Portfolio Management | Nonlinear regression model Network of connected nodes Training data and desired output data Enabling deep learning capabilities |
Asset Classification, Rebalancing | Clustering of data into groups with similar characteristics Number of clusters are defined by the algorithms It is primarily used in asset classification It includes several unique algorithms and methods |
Fundamental Analysis for Testing | Techniques used to process textual and audio data Useful to perform sentiment analysis on investors It is widely used during fundamental analysis (analysis of organisation reports and latest feeds) |
Forecasting, Diversification | Decision tree classifies units based on the features Random forests are the average of the outputs of multiple decision trees Mostly used in asset classification and forecasting Classification is done by branching a logical tree from root to leaves |
In summary, while AI holds great potential to revolutionise processes in all aspects of business, including treasury management, there are serious ethical dilemmas to consider when deploying the technology. Experts in this field are continually looking for ways to address these issues, but feedback and interaction from corporate treasurers is always welcome to help shape a more powerful, equitable, future for AI.